Independent Study
Independent Study Student in the Department of Geography and Planning Appalachian State University, Boone, NC
From my previous URA project, this Independent Study continues with the existing project, which is studying Social Determinants of Health (SDoH) along with other health indexes. For the Fall 2020 semester, I was honored to continue working with Dr. Maggie Sugg and Lauren Andersen, along with Dr. Jennifer Runkle of the North Carolina Institute for Climate Studies.
Using the SDoH data from the URA project, this Independent Study follows the Principal Component Analysis (PCA) data of the SDoH variables and uses a relatively new health metric. In recent research, the Index of Concentration at Extremes (ICE) has been introduced to measure disadvantaged and advantaged populations. The objective of this study evolved to analyzing these health metrics as to which one is a better predictor of Life Expectancy at a sub-county level. The SDoH index used 66 variables that were Z-score standardized for the PCA analysis, and these variables were acquired from the URA project. The ICE index combines 6 factors to create three metrics - Income, Race, and Income + Race. Spatial analysis was required to determine the research questions of the spatial patterns of the metrics and which one is the best predictor. ArcGIS Pro and GeoDa were used to assess the heterogeneity and identify locations that could benefit from targeted public health interventions to address underlying health disparities. Results indicate that both metrics can determine spatial patterns of inequities for LE in NC; however, the metrics vary in predictability performance.
Methods and Processes - What I Have Learned
One of the main goals of this Independent Study was to write a manuscript for publication.
The manuscript will be submitted for publication upon competition.
-
Conducting Spatial Analysis involved learning how to use the Spatial Regression tools in ArcGIS Pro. I learned how to conduct an Ordinary Least Squares (OLS) spatial regression along with interpreting the results.
-
Interpreting results requires evaluating the Adjusted R-Squared value (assessing how much variation in the dependent variable is explained), Coefficients, VIF Values (Variable Inflation Factor, assessing variable redundancy), AIC Values (Akaike's Information Criterion, assessing model performance)
-
-
The OLS regression also involved conducting the Global Moran's I test for assessing spatial autocorrelation. From previous work, I understood how to conduct the Moran's I test within ArcGIS Pro.
-
The OLS regression was used on each ICE metric (total 3), the SDoH Components 1-16 combined (total 1), and the calculated Vulnerable field from the SDoH PCA results (total 1).
-
The acquired Life Expectancy data did not account for all Census tracts, and a logarithmic transformation was conducted on the Life Expectancy data. The transformed Life Expectancy variables were used as the Dependent Variable in the OLS regression.
-
To assess the validity of the results, OLS regression was conducted using GeoDa. The same variables were selected and results showed a similarity between the two program models.
-
From the GeoDa OLS spatial regression results, it was decided to use the Spatial Error spatial regression model as the final regression choice.
-
Results were furthered analyzed in the software, R.
SDoH Index
-
SDoH related factors (U.S. Census Bureau, ACS)
-
Public and Health Resource (NC One Map and HIFLD data)
-
Health Professional Shortage Area (Health Resources and Service Administration)
-
Encompass Employment, Education, Household Conditions, Household Income, Work Transportation, Health Factors, and the distribution of public and health services.
ICE Index
-
ICE Income - Household Income Less than $10,000 to $25,000 and Household Income $100,000 or More (U.S. Census Bureau, ACS)
-
ICE Race - Non-Hispanic White population and Non-Hispanic Black population
-
ICE Income + Race - Non-Hispanic Black Households with Less than $10,000 to $25,000 and Non-Hispanic White Households with $100,000 or more
Life Expectancy
-
Census-tract level data (CDC, National Center for Health Statistics)
Completed Maps
Spatial Regression | Spatial Analysis Results
The tables below include the Spatial Regression Model results from ArcGIS Pro, GeoDa, and R. It details the Independent Variables, along with the OLS and Spatial Error result factors used to indicate reliability and accuracy.
The Spatial Error model proved to have the highest R-squared values, as confirmed by using the software, R. The SDoH components have the highest R-squared model of nearly 57%, and the combined ICE metrics having a predictability of just over 54%.
The results from R show similar results, with the SDoH components having nearly 56% predictability.
This study shows that the SDoH and ICE metric have comparable results in predicting life expectancy. It emphasizes that socioeconomic factors do impact life expectancy. However, factors such as income and race still are prevalent, determinative factors affecting life expectancy. In the field of public health, this study proves that using the ICE metric can show the health of a community nearly as much as the SDoH model.
Using the ICE metric can be more beneficial to the public health field because it requires fewer variables to acquire and can be generated faster to measure a community's health. The results also indicate that factors such as income and race, ranging from privilege to deprivation, is nearly as significant as incorporating education, employment, income, household conditions, and health factors. The SDoH model was created based on known research methodology that measures vulnerability and deprivation. The results of this study indicate that simpler measures like ICE capture underlying health disparities. Existing research emphasizes the use of the SDoH, however, this study indicates the feasibility of the ICE metric since the predictability is as high as it is.
Most Recent Project Work
Southeastern Division of the Association of American Geographers (SEDAAG)
2020 Virtual Conference (SEDAAG)
While completing the spatial analysis and writing the research manuscript, the opportunity for the SEDAAG conference became available. To participate, the requirements included writing an abstract and creating a poster for an Undergraduate Poster Competition.
During this process, I was able to write a stronger abstract that defined my project and the latest results. I also learned how to create a research poster showcasing my work.

Included is a PDF file of additional research information.
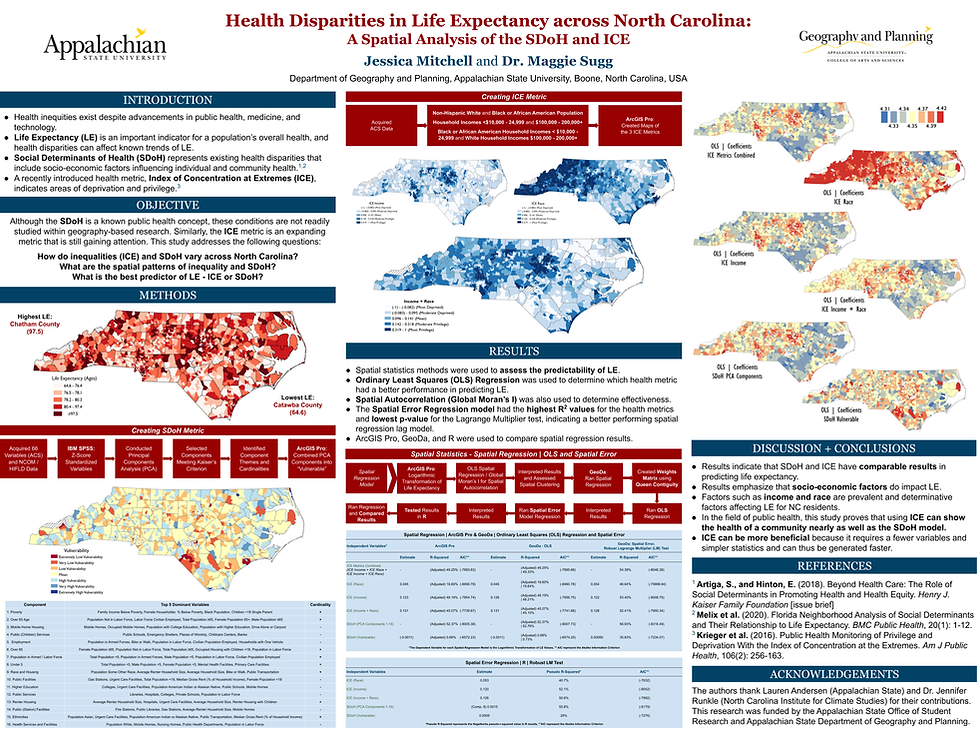
Included is a PDF file of the SEDAAG poster.
Manuscript Maps
Below are the maps (along with a PDF version) for the final maps used in the manuscript submission. Upon writing the manuscript and following journal publication rules, it was necessary to combine the SDoH and ICE maps and create a bivariate map of the two metrics. The bivariate map includes combining the most vulnerable fields from the SDoH and ICE metrics.
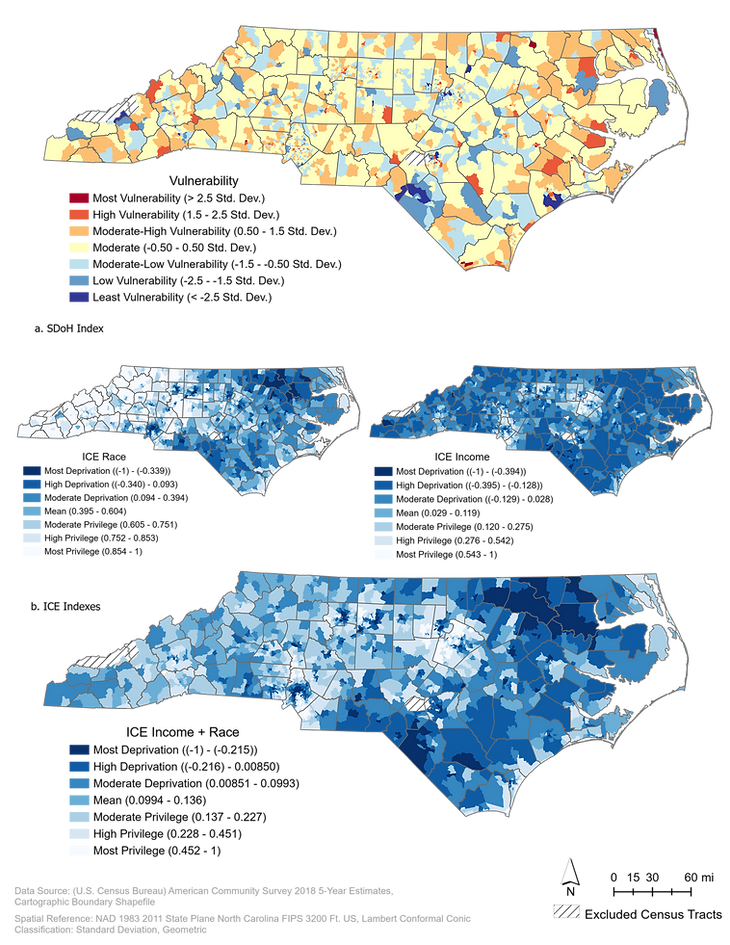
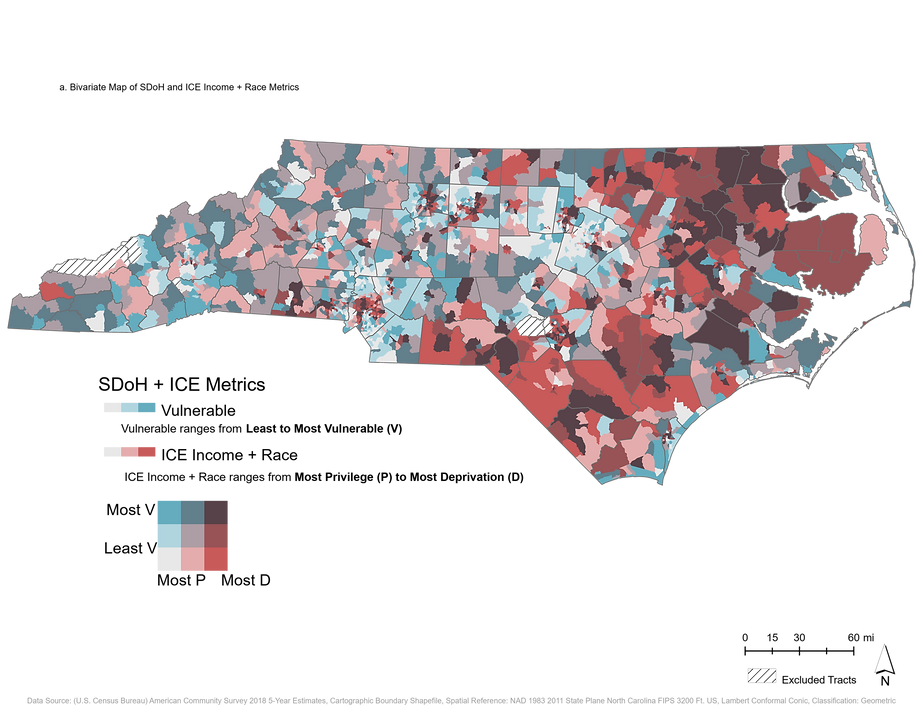
As of December 2020, the manuscript was submitted for publication
awaiting approval and review.